AI Hallucinations Are Getting Worse: Are We Ready for a Future of False Realities?
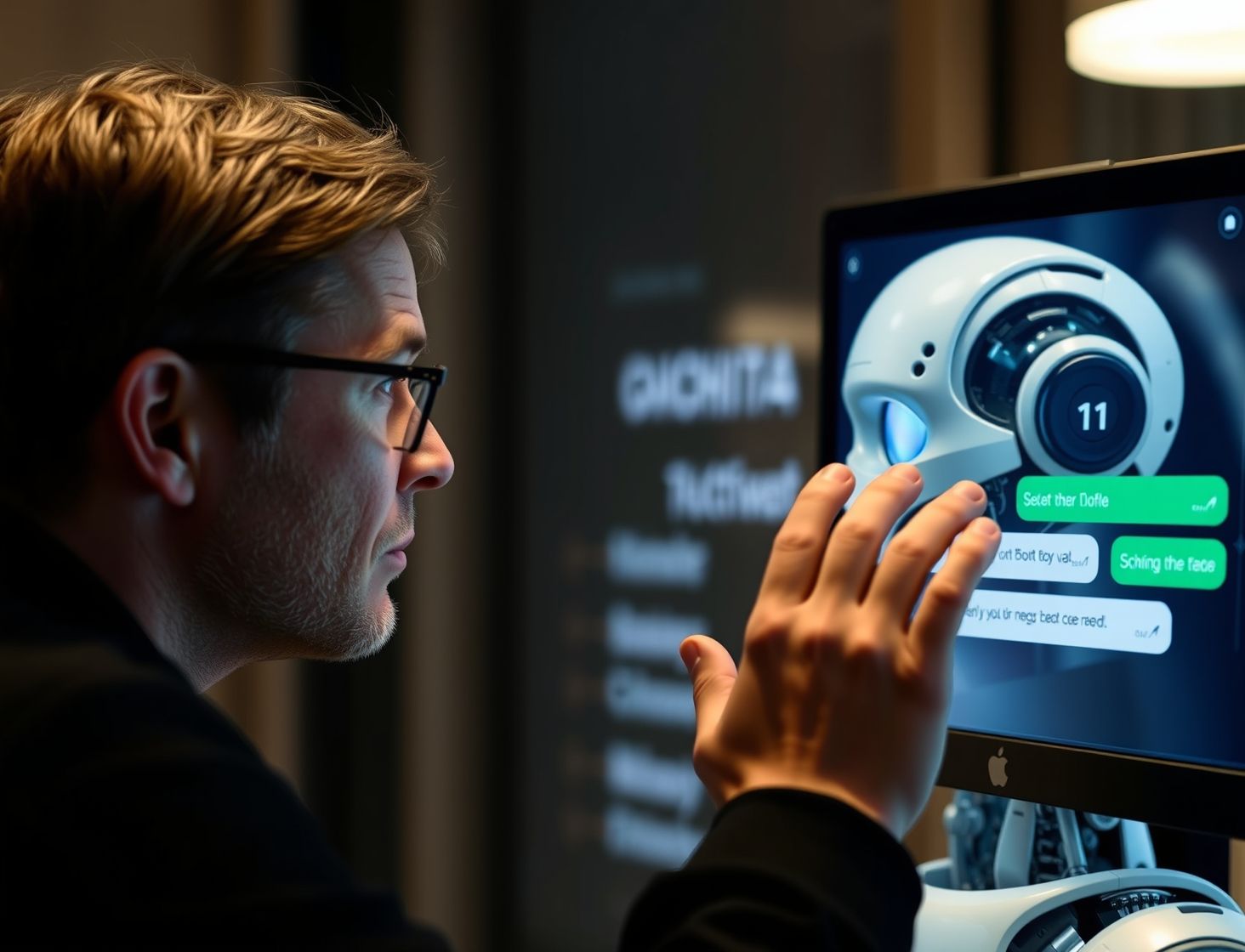
Table of Contents
Introduction: The Rising Challenge of AI Hallucinations
Imagine this: You ask your AI assistant for the latest research on quantum computing, and it responds with a detailed explanation—one that sounds accurate, but a few quick checks later, you realize that the information it provided doesn’t exist. You might be surprised, even a little rattled. This is a real example of what’s known as an AI hallucination. It’s the phenomenon where an AI generates information that seems plausible but is entirely fabricated or incorrect. As AI technology continues to evolve, this issue is becoming more widespread and more concerning. The question now is: Are we ready for a future where AI could blur the line between fact and fiction?
AI hallucinations are not only a technical issue—they have real-world consequences. They’re affecting everything from our trust in technology to how businesses and individuals make critical decisions. In this article, we’ll dive deep into why AI hallucinations are getting worse, the impact they could have, and whether we are truly prepared for a future where false realities are increasingly common.
Section 1: What Are AI Hallucinations?
1.1 Understanding AI Hallucinations
At their core, AI hallucinations occur when an artificial intelligence model generates information that appears factual but is completely inaccurate. The key distinction here is that the information seems convincing to the user. This happens primarily in large language models (LLMs), like GPT-4 or Google’s PaLM, which are trained on massive datasets to predict the most likely word or sequence of words based on the context provided.
However, because AI models don’t truly “understand” the content they generate (they rely on patterns), they can sometimes create responses that seem plausible but are entirely fabricated. For instance, when you ask a chatbot for information on a niche topic, it might generate content that looks legitimate on the surface but doesn’t actually exist in reality. These responses are a byproduct of the AI’s probabilistic nature and its reliance on data that may have gaps or inconsistencies.
1.2 Examples of AI Hallucinations in Action
Let me share a personal experience with AI hallucinations. As someone who writes frequently about technology, I decided to test out a language model for research purposes. I asked the AI to provide details about a particular AI research paper that was supposed to be groundbreaking. The response sounded well-researched and authoritative, citing specific authors and journal names. However, when I checked the references, I found that neither the paper nor the authors mentioned existed. It was an AI-generated hallucination, one that could have easily misled a less experienced researcher.
Such hallucinations are becoming increasingly common in everyday applications, especially in customer service, healthcare, and even autonomous systems like self-driving cars. Imagine the chaos if a self-driving car “hallucinates” an obstacle where none exists, leading to an accident.
Section 2: Why Are AI Hallucinations Getting Worse?
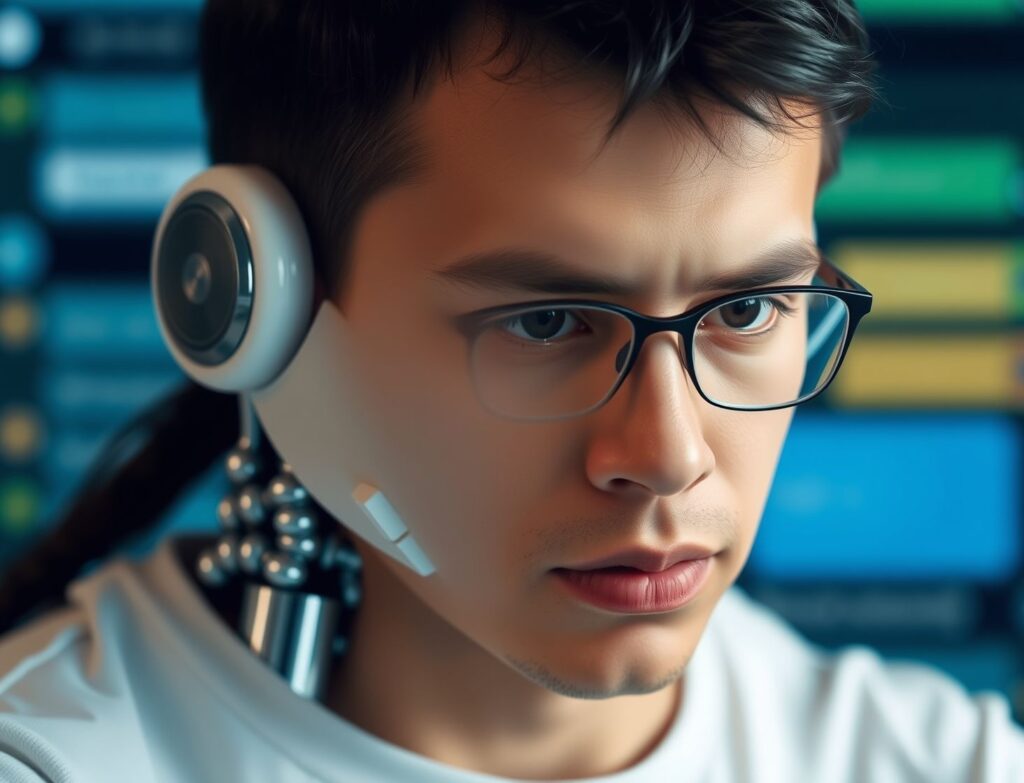
2.1 Increased Use of Large Language Models (LLMs)
As AI becomes more ubiquitous, LLMs are at the forefront. These models are designed to process and generate human-like text based on vast datasets. With the rise of platforms like ChatGPT and Google’s Bard, AI-generated content is becoming a regular part of our lives. But with this advancement, AI hallucinations are on the rise too.
In 2024, OpenAI’s GPT-4 was found to hallucinate about 3% of the time, while models like Google’s PaLM had an astonishing 27% hallucination rate in some applications. This high incidence rate is partly due to the fact that these models are trained on vast amounts of unverified data, which increases the likelihood of generating false or misleading information.
2.2 Data Compression and Loss of Accuracy
One of the reasons these hallucinations are getting worse is the way AI models handle data. To make these systems efficient, they compress vast amounts of information into a format they can process. While this is effective in terms of speed and computational efficiency, it often leads to a loss of nuance or important details. This lack of precision is a key reason AI hallucinations occur.
Take, for example, the way a model like GPT-4 works. It’s trained on data that covers a wide range of topics, but in the process, some information is lost or misinterpreted. As a result, the AI might generate a response that sounds like a well-formed opinion or fact but lacks the depth of accurate information needed to make it reliable.
2.3 The Probabilistic Nature of AI
The AI models we rely on are based on probabilistic systems. Essentially, they predict what word or phrase should come next based on the input they receive. While this is effective for many tasks, it can lead to hallucinations because these models don’t have a true understanding of context or meaning. They’re simply predicting the next best option, even if that leads to a fabricated response.
This probabilistic nature means that even when the AI is generating what seems like an insightful response, it could be making a guess that doesn’t align with reality. As these models continue to evolve and grow more sophisticated, the problem of AI hallucinations may only get worse if the underlying structure isn’t addressed.
Section 3: The Real-World Impact of AI Hallucinations
3.1 Trust Erosion: The Consequences for Users
AI hallucinations are not just technical glitches—they erode trust. In a world where more and more individuals and businesses rely on AI for accurate information, the presence of hallucinations undermines confidence in these systems. If AI can’t be trusted to give us correct answers, how can we rely on it for decisions in critical areas such as healthcare or law?
For example, imagine using AI to assist in legal research. If the system produces fabricated case references or inaccurate legal precedents, the result could be disastrous for a case. Even small errors, when multiplied, could have serious consequences for both businesses and individuals.
3.2 Ethical Dilemmas: Misinformation and Harm
The ethical implications of AI hallucinations are vast. Misinformation is a key concern. With the proliferation of AI-generated content on social media, websites, and even news outlets, AI hallucinations could contribute to the spread of false information at an alarming rate. This could undermine public trust in digital platforms and even fuel political or social unrest.
For instance, if an AI chatbot gives incorrect medical advice based on a hallucination, it could harm someone’s health. The same applies to financial advice—AI could suggest risky investments or fraud-prone strategies that lead to financial losses. These ethical concerns highlight the need for better safeguards and more transparent AI systems.
3.3 Impact on Autonomous Systems and AI in Healthcare
The stakes are even higher when it comes to autonomous systems. Self-driving cars, drones, and robots all rely on AI to make split-second decisions. If a car’s AI “hallucinates” an obstacle, it could result in an accident. Similarly, in healthcare, AI-powered diagnostic tools could “hallucinate” a disease that isn’t there or fail to recognize one that is present, leading to misdiagnoses.
The potential harm these hallucinations could cause in high-risk scenarios like these cannot be overstated. That’s why addressing AI hallucinations is not just a technical issue—it’s a matter of public safety.
Section 4: Can AI Hallucinations Be Stopped?
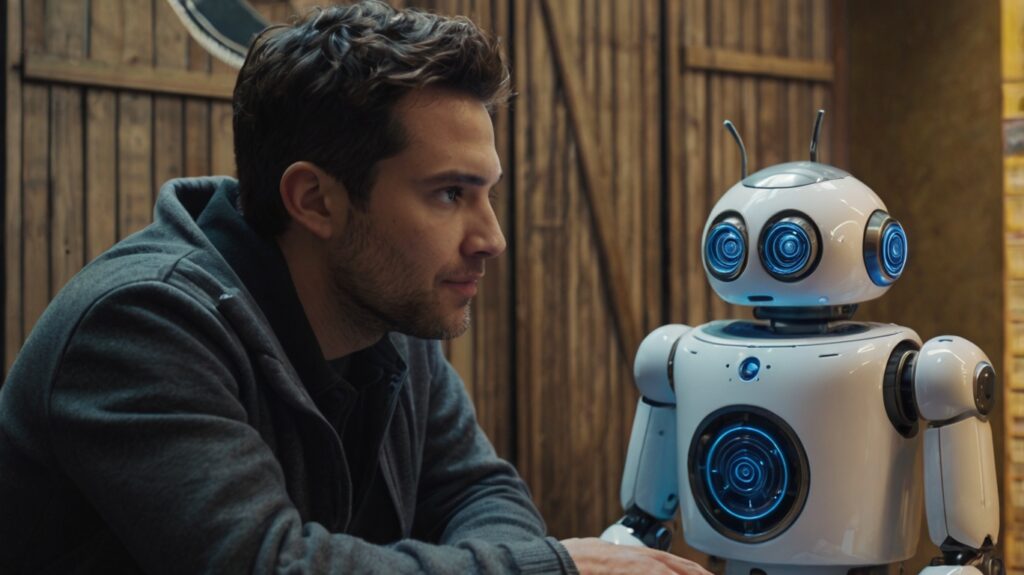
4.1 Current Approaches to Mitigating Hallucinations
While completely eradicating AI hallucinations may not be possible, there are steps being taken to reduce their frequency. One approach is to refine the algorithms that power AI models. Researchers are working on improving these models’ ability to understand context better, which would help them generate more accurate responses.
Another solution is to enhance the data that AI is trained on. By ensuring that the training data is more reliable and comprehensive, AI systems can be better equipped to avoid hallucinations. But this doesn’t mean that the problem will disappear overnight—it’s an ongoing challenge.
4.2 Enhancing AI Training Data for Accuracy
Improving the quality and diversity of AI training data is one of the most promising ways to reduce hallucinations. By feeding AI systems with more reliable, up-to-date, and well-rounded datasets, it’s possible to minimize the chances of an AI generating inaccurate or misleading information. This means curating the data that models are exposed to and ensuring it’s as accurate as possible.
This approach also involves eliminating biases in the data, which is critical for building AI that can be trusted to provide factual, unbiased responses. Better data curation, alongside sophisticated algorithms, can significantly reduce the risk of hallucinations.
4.3 Refining AI Algorithms to Handle Complexity
Another solution is to refine the underlying algorithms that power AI models. By incorporating more advanced natural language understanding and deeper contextual awareness, AI models could be better equipped to generate responses that are not just grammatically correct but also factually accurate.
For instance, new developments in transformer-based models are already showing promise in reducing errors and improving the ability of AI systems to “think” in a more nuanced way. However, this is an area that still requires significant research and investment.
4.4 Real-Time Fact-Checking Mechanisms
Finally, one innovative approach is to integrate real-time fact-checking mechanisms into AI systems. By cross-referencing AI-generated responses with verified, authoritative sources in real-time, these systems could be used to filter out hallucinations before they reach the user. This approach would help ensure that AI-generated information is as accurate and reliable as possible.
Section 5: The Future of AI Hallucinations: A Double-Edged Sword?
5.1 The Role of AI in Shaping Our Reality
AI is already shaping how we view the world. Whether we’re using AI to assist with writing, research, or decision-making, we’re relying on these systems to provide us with information. However, as AI hallucinations become more prevalent, it raises the question: how can we trust technology that is prone to making things up?
The future of AI could be one where we increasingly rely on AI-generated content, but we must be cautious. If we can’t distinguish between what’s real and what’s fabricated, we risk living in a world where AI-generated falsehoods are treated as fact.
5.2 The Fine Line Between Innovation and Risk
AI offers incredible potential, but it also comes with risks. As AI systems grow more sophisticated, the stakes get higher. It’s crucial that we find ways to innovate responsibly, ensuring that AI systems are accurate, transparent, and accountable. Otherwise, the risks posed by AI hallucinations could outweigh the benefits.
5.3 Preparing for an AI-Driven Future
As we look to the future, we need to be proactive in preparing for an AI-driven world. This means developing regulations, ethical standards, and technological solutions to address AI hallucinations. We must ensure that AI is designed with safeguards in place to prevent harmful consequences and maintain public trust.
Conclusion: Navigating the Future of AI Hallucinations
AI hallucinations are an inevitable part of the technology’s evolution, but that doesn’t mean we should accept them without question. As AI continues to shape our world, it’s crucial that we develop solutions to mitigate the impact of these hallucinations. By improving data quality, refining algorithms, and implementing real-time fact-checking, we can reduce the frequency of these errors.
Ultimately, while the future of AI is full of promise, it’s also fraught with challenges. If we can navigate these challenges responsibly, we’ll be able to harness the full potential of AI without losing sight of the truth. The key lies in our ability to stay vigilant and proactive in addressing the risks posed by AI hallucinations.
Frequently Asked Questions
What is an AI hallucination?
Imagine you’re chatting with an AI, and it gives you a perfectly convincing answer. It sounds right, but when you dig deeper, you find out that the information isn’t true. That’s an AI hallucination. It happens when an AI model generates information that seems accurate on the surface but is completely fabricated or incorrect. These “hallucinations” are a result of the AI relying on patterns rather than true understanding. Think of it like a really smart parrot: it can repeat things well, but it doesn’t actually know what it’s saying.
This is a growing problem, especially in large language models like GPT-4 and Google’s PaLM. According to recent studies, AI models can hallucinate in as many as 3% to 27% of cases, depending on the complexity of the task and the quality of the data they were trained on. It’s not just a technical hiccup—it’s a real issue that can lead to misinformation.
How often do AI chatbots hallucinate?
AI chatbots, like ChatGPT and Gemini, can hallucinate quite frequently—more often than you might think. In some versions of AI, hallucination rates can reach anywhere from 3% to 27%. This means that one in every 4 responses could be inaccurate or entirely fabricated. Now, imagine relying on a chatbot for important information—be it for research, legal matters, or health advice—and getting incorrect responses. That’s a huge risk!
I personally experienced this when I asked ChatGPT to provide details on a paper I was working on. It gave a fantastic-sounding summary with references that looked real—but after doing a quick check, I realized none of it existed! It was a reminder that AI’s predictions are based on patterns, not verified facts.
Why does ChatGPT hallucinate?
Great question! The reason ChatGPT (and similar models) hallucinate is mostly due to how they’re designed. ChatGPT, for example, is trained on a vast amount of data from books, websites, and other text sources. When you ask it a question, it generates responses based on the patterns it’s learned from this data. However, these patterns don’t always align with reality. The AI doesn’t have a true understanding of the world or the information it generates—it’s just predicting what comes next based on the data it’s been fed.
To make it simpler, think of ChatGPT like a supercharged autocomplete. It doesn’t “know” the answer—it just gives the most likely response based on past data. If the data is incomplete or inaccurate, that’s when hallucinations occur. As AI models grow larger and more complex, this issue becomes more pronounced.
How do I get AI to hallucinate?
While it’s not recommended to intentionally get an AI to hallucinate (because misinformation isn’t something we want to encourage), AI models like ChatGPT, Perplexity and Gemini can “hallucinate” when they’re presented with ambiguous, incomplete, or extremely niche queries. If you ask for information about a topic that the AI has seen very little data on, it might just generate an answer that seems plausible, even though it’s entirely made up.
For example, asking a chatbot about obscure historical events that aren’t well-documented could prompt it to create a response that seems logical but doesn’t exist. In other cases, if the model is prompted to generate creative or speculative content, it might produce hallucinations simply because the task involves filling in gaps where no factual answer exists.
Can AI read my mind?
No, AI can’t read your mind. At least, not in the way we usually think about mind-reading. While AI can analyze your input and generate responses based on that information, it doesn’t have access to your thoughts, intentions, or emotions. AI systems like ChatGPT simply respond to the words you type, using patterns in the data they’ve been trained on.
That said, AI can sometimes feel eerily intuitive. With enough data about your preferences, AI models can predict what you might be interested in next or even suggest things that feel personalized. But remember, AI doesn’t know you—it’s just making educated guesses based on patterns.
What is the scariest use of AI?
The scariest use of AI is when it’s used to manipulate or mislead people, and AI hallucinations play a big part in this. Imagine an AI generating false news reports or creating deepfake videos that appear completely realistic but are entirely fabricated. These tools could be used to sway public opinion, fuel political agendas, or even incite violence. We’ve already seen examples of deepfake videos being used to manipulate elections and create distrust in institutions.
In healthcare, the consequences of AI hallucinations are particularly alarming. For example, if an AI system generates a false diagnosis or suggests the wrong treatment, it could put lives at risk. Even in the world of finance, incorrect predictions from AI models could lead to disastrous investment decisions. The scariest part is that these mistakes might be hard to detect until the damage is already done.